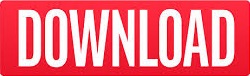

fns, specifies what to do with each column. To demonstrate this new flexibility in a more useful situation, let’s take a look at quantile(). cols, specifies which columns you want to iterate over, and the second argument.
#Dplyr summarize 3 columns in r code
This is a big change to summarise() but it should have minimal impact on existing code because it broadens the interface: all existing code will continue to work, and a number of inputs that would have previously errored now work. In Microsoft Excel, often the most popular/frequent way to do grouped calculations (counting, aggregation, etc) is to look at this row's group variable(s) and compare with the previous row's group variable(s) to see if anything has changed, and use or reset as appropriate. three categories in column 1, are represented in the light grey, blue and green rows. To put this another way, before dplyr 1.0.0, each summary had to be a single value (one row, one column), but now we’ve lifted that restriction so each summary can generate a rectangle of arbitrary size. (previous answer) I recommend against the use of what I call 'Excel grouping'. & dplyr functions work with pipes and expect tidy data.
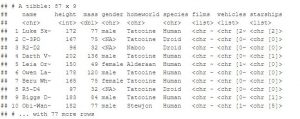
Multiple columns are combined into one value column with a key column keeping track. (This isn’t very useful when used directly, but as you’ll see shortly, it’s really useful inside of functions.) Apply summary functions to columns to create a new table of summary statistics. arrange count filter select and rename summarise and summarize. Df %>% group_by ( grp ) %>% summarise ( tibble ( min = min ( x ), mean = mean ( x ))) #> `summarise()` ungrouping output (override with `.groups` argument) #> # A tibble: 2 x 3 #> grp min mean #> * #> 1 1 -2.69 -0.843 #> 2 2 -2.73 -0.434
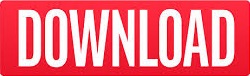